Predictive analytics is all about using data to predict future outcomes. It helps businesses make better decisions. Machine learning plays a big role in predictive analytics. It can process large amounts of data, find patterns, and make accurate predictions.
Let’s explore how machine learning and predictive analytics work together.
What Is Predictive Analytics?
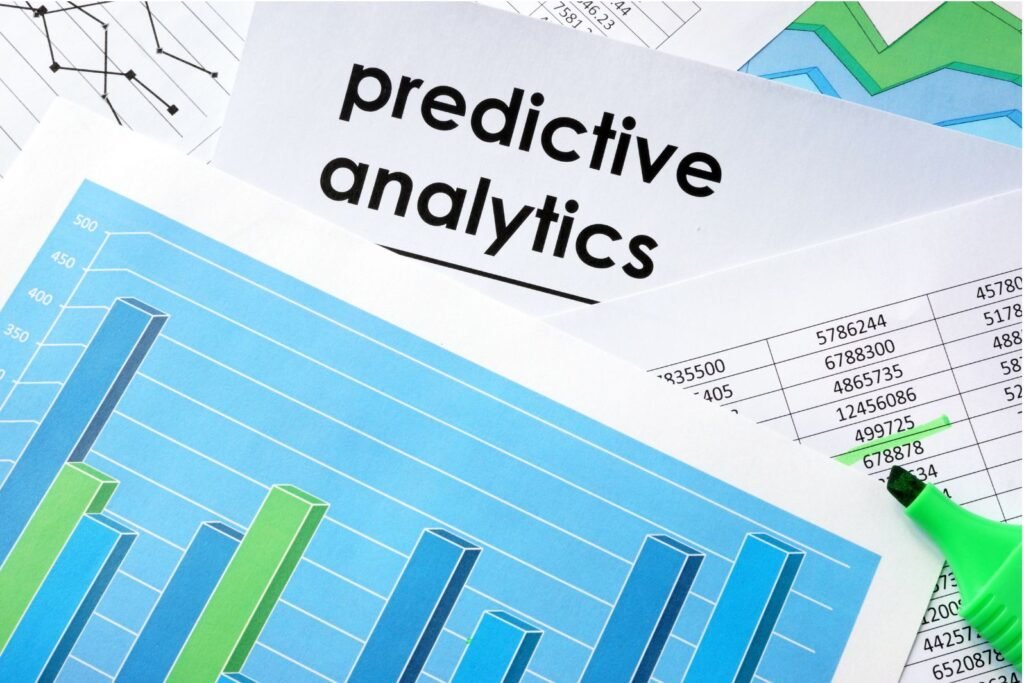
Predictive analytics uses data to predict what might happen in the future. It looks at past trends and patterns to make these predictions. Businesses use predictive analytics to plan better, reduce risks, and improve performance.
For example:
- An online store can predict what products a customer might buy.
- Banks can spot signs of fraud before it happens.
- Hospitals can predict which patients might need extra care.
What Is Machine Learning?

Machine learning is a type of artificial intelligence. It teaches computers to learn from data. Instead of following fixed instructions, machines improve their performance by studying patterns in the data.
For example:
- A music app learns your favorite songs and suggests similar ones.
- An email service identifies spam messages and keeps them out of your inbox.
How Does Machine Learning Help Predictive Analytics?
Machine learning makes predictive analytics more powerful. Here’s how:
1. Handling Big Data
Businesses generate a lot of data every day. This data comes from websites, apps, social media, and customer interactions. It is too much for humans to process manually.
Machine learning tools can quickly process this data. They organize it and find useful patterns. This makes it easier for businesses to understand what is happening and what might happen next.
2. Identifying Patterns
Machine learning is great at spotting patterns in data. For example:
- A machine learning model can analyze shopping habits. It can find out what time of year people buy certain products.
- It can also identify which customers are likely to stop using a service.
These patterns help businesses create better strategies to keep customers happy.
3. Making Accurate Predictions
Machine learning models learn from past data. The more they learn, the better their predictions become.
For instance:
- A machine learning model can predict how many sales a business will have next month.
- It can also predict when a machine in a factory might break down.
Accurate predictions save time and money.
4. Adapting to New Data
One of the best things about machine learning is that it keeps improving. When new data comes in, machine learning models update themselves. They adapt to changes and stay accurate.
For example:
- A ride-sharing app can adjust its predictions for busy times during unexpected events, like a sudden rainstorm.
Examples of Machine Learning in Predictive Analytics:
1. Healthcare
In healthcare, machine learning helps doctors predict health risks. For example:
- It can analyze patient records to predict who might develop certain diseases.
- It can also help hospitals predict how many patients they might need to care for during flu season.
This helps doctors provide better care and hospitals prepare for emergencies.
2. Retail
Retailers use machine learning to predict customer behavior. For example:
- A store can predict which products will sell best during the holidays.
- It can also predict when customers might stop shopping with them.
This helps stores stock the right products and create offers to keep customers coming back.
3. Finance
Banks and financial companies use machine learning to predict risks. For example:
- It can predict which customers might miss loan payments.
- It can also spot unusual transactions that could be fraud.
This helps banks make better decisions and keep their customers safe.
4. Manufacturing
In factories, machine learning predicts equipment failures. For example:
- It can analyze how machines are working and predict when they might need maintenance.
This prevents breakdowns and keeps production running smoothly.
5. Sports
Sports teams use machine learning to predict player performance. For example:
- It can analyze player statistics and predict who might perform well in the next game.
This helps teams make better decisions during matches.
Types of Machine Learning in Predictive Analytics:
1. Supervised Learning
In supervised learning, the model learns from labeled data. This means the data has clear inputs and outputs.
For example:
- A model learns that certain weather conditions (input) cause a traffic jam (output). It can then predict traffic jams based on future weather conditions.
2. Unsupervised Learning
In unsupervised learning, the model works with unlabeled data. It finds hidden patterns on its own.
For example:
- A model can group customers with similar shopping habits. Businesses can then create special offers for each group.
3. Reinforcement Learning
In reinforcement learning, the model learns through trial and error. It tries different actions and learns from the results.
For example:
- A robot learns the best way to move through a maze by trying different paths and learning from mistakes.
Benefits:
1. Speed
Machine learning processes data much faster than humans. This allows businesses to make quick decisions.
2. Accuracy
Machine learning models make fewer mistakes when trained properly. This means businesses can trust the predictions.
3. Cost Savings
By predicting risks and solving problems early, machine learning saves businesses time and money.
4. Scalability
Machine learning can handle huge amounts of data. As businesses grow, machine learning tools can grow with them.
Challenges of Using Machine Learning in Predictive Analytics:
1. Data Quality
Machine learning models need good data to make accurate predictions. If the data is incomplete or incorrect, the predictions will be wrong.
2. Complexity
Setting up machine learning models can be complex. It requires skilled people and the right tools.
3. Privacy Concerns
Using customer data comes with privacy concerns. Businesses must follow rules to protect sensitive information.
4. Cost of Implementation
Machine learning systems can be expensive to set up. Smaller businesses may find it hard to afford them.
The Future of Machine Learning in Predictive Analytics:
Machine learning will play an even bigger role in predictive analytics in the future. With advancements in technology, models will become faster, smarter, and easier to use.
Here are some trends to watch:
- Real-Time Predictions: Businesses will use machine learning to make instant decisions. For example, predicting traffic and adjusting delivery routes on the spot.
- Integration with AI: Predictive analytics will combine with other AI technologies to provide more insights.
- More Accessible Tools: Tools for predictive analytics will become cheaper and easier to use, helping more businesses benefit.
Conclusion:
Machine learning is a key part of predictive analytics. It helps businesses process large data sets, find patterns, and make accurate predictions. From healthcare to finance, it is transforming industries and helping organizations stay ahead.
Although there are challenges, the benefits far outweigh them. As technology improves, machine learning in predictive analytics will only become more powerful. Businesses that embrace it now will be better prepared for the future.